Information Coefficient Ic Definition Example And Formula
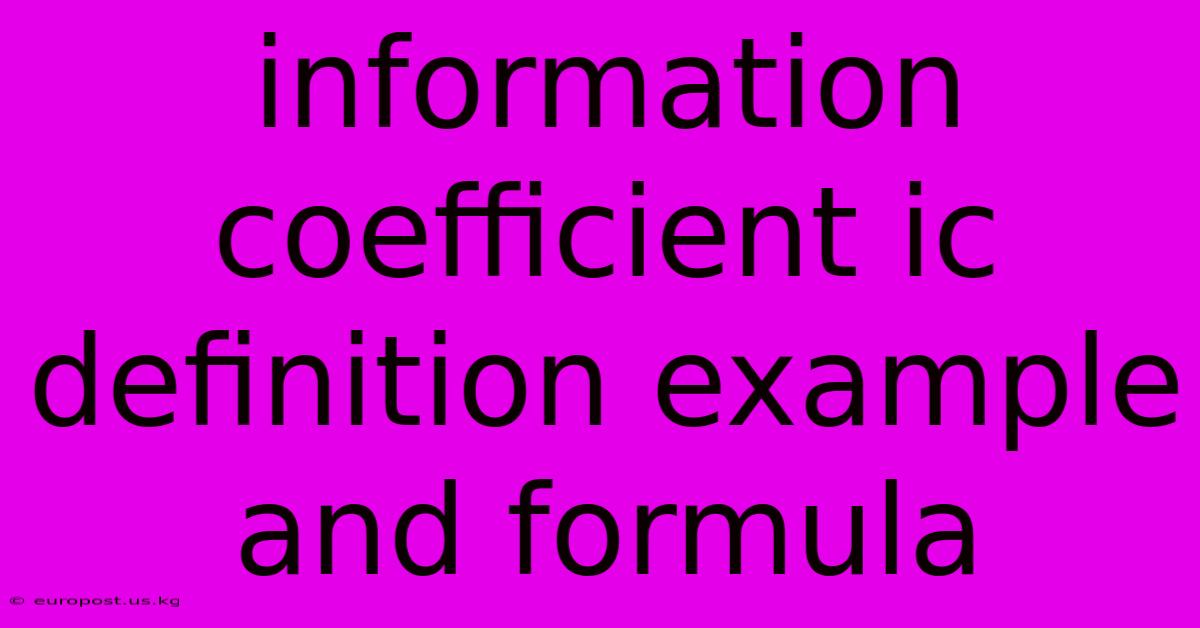
Discover more in-depth information on our site. Click the link below to dive deeper: Visit the Best Website meltwatermedia.ca. Make sure you don’t miss it!
Table of Contents
Unveiling the Secrets of Information Coefficient (IC): Exploring Its Pivotal Role in Quantitative Finance
Introduction: Dive into the transformative power of the Information Coefficient (IC) and its profound influence on evaluating predictive models in quantitative finance. This detailed exploration offers expert insights and a fresh perspective that captivates professionals and enthusiasts alike.
Hook: Imagine if the secret to accurately predicting market movements could be encapsulated in a single, transformative metric—the Information Coefficient (IC). Beyond being just a statistical measure, the IC is the invisible force that drives confidence in forecasting models, allowing investors to separate signal from noise and make more informed decisions.
Editor’s Note: A groundbreaking new article on the Information Coefficient (IC) has just been released, uncovering its essential role in shaping effective quantitative trading strategies.
Why It Matters: The Information Coefficient is the cornerstone of evaluating the predictive power of any quantitative model used in finance, from stock selection to risk management. This deep dive reveals its critical role in assessing signal strength, understanding model limitations, and ultimately, maximizing investment returns. A robust understanding of IC is crucial for anyone involved in quantitative finance, from portfolio managers to data scientists.
Inside the Article
Breaking Down the Information Coefficient (IC)
Purpose and Core Functionality: The Information Coefficient (IC) quantifies the correlation between a predictive model's output (a signal, forecast, or rank) and the actual realized outcome. It measures the strength of the relationship, indicating how well the model predicts future events. Unlike other metrics, IC focuses on the rank correlation, not the linear correlation. This makes it robust against non-linear relationships between the prediction and the outcome. A higher IC suggests a more accurate and valuable predictive model.
Role in Ranking and Selection: The IC is particularly useful when dealing with ranked data. In many financial applications, the exact magnitude of a prediction is less important than its relative rank compared to other potential investments. For example, if a model accurately predicts which stocks will perform best relative to each other, it has high IC even if the predicted magnitude of the returns isn't perfectly accurate.
Impact on Sharpe Ratio and Portfolio Construction: The IC is directly related to the Sharpe ratio, a crucial measure of risk-adjusted return. A higher IC generally translates to a higher Sharpe ratio, indicating better risk-adjusted performance for a portfolio constructed using the model's predictions. Therefore, IC helps determine which models will contribute most effectively to portfolio construction and optimization.
Exploring the Depth of Information Coefficient (IC)
Opening Statement: What if there were a metric so integral it could objectively assess the predictive value of any financial model? That’s the IC. It shapes not only our understanding of model performance but also the confidence with which we deploy those models in real-world trading strategies.
Core Components: The Formula and its Interpretation
The IC is calculated using the Spearman rank correlation coefficient, which measures the monotonic relationship between two ranked variables. The formula is:
IC = ρ<sub>s</sub> = 1 − [6Σd<sub>i</sub>² / {n(n² − 1)}]
Where:
- ρ<sub>s</sub> represents the Spearman rank correlation coefficient (which is the IC).
- d<sub>i</sub> is the difference between the ranks of the predicted values and the actual values for each observation i.
- n is the number of observations.
The IC ranges from -1 to +1:
- IC = +1: Perfect positive correlation. The model perfectly predicts the rank order of outcomes.
- IC = 0: No correlation. The model’s predictions have no predictive power.
- IC = -1: Perfect negative correlation. The model perfectly predicts the reverse rank order of outcomes (i.e., it's perfectly inversely predictive).
In-Depth Analysis: Real-World Examples
Consider a stock selection model aiming to predict the top 10 performing stocks in a given year. The model assigns a score to each stock, and these scores are then ranked. The actual performance of each stock is also ranked based on its return. The IC measures the correlation between the model's predicted rank and the actual rank. If the IC is high (e.g., 0.3 or higher), it indicates that the model is successfully identifying higher-performing stocks relative to lower-performing stocks.
Another example involves a credit scoring model predicting defaults. The model ranks borrowers based on their predicted likelihood of default. The actual default status (default or no default) is also ranked. A high IC suggests the model is effective in identifying risky borrowers.
Interconnections: IC and Other Performance Metrics
While the IC is a powerful standalone metric, it's often used in conjunction with other measures to provide a holistic assessment of a model's performance. For instance, the IC can be combined with the model's turnover (the frequency of changes in the portfolio) to assess the trade-off between predictive accuracy and transaction costs.
FAQ: Decoding the Information Coefficient (IC)
What does the Information Coefficient (IC) do? It measures the predictive power of a ranking model, showing how well it predicts the relative order of outcomes, not their exact values.
How does it influence trading decisions? A higher IC indicates a more reliable model, enabling more confident investment choices and portfolio construction.
Is it always relevant? Yes, especially in scenarios where relative ranking is crucial (e.g., stock selection, credit scoring).
What happens when the IC is low? A low IC signals that the model needs improvement or should be discarded, as its predictions offer limited value.
Is the IC influenced by market conditions? The IC can be affected by market regimes, emphasizing the need for robust models adaptable across different market environments.
Practical Tips to Master the Information Coefficient (IC)
Start with the Basics: Understand the Spearman rank correlation and its application to financial data. Step-by-Step Application: Practice calculating the IC using sample datasets. Learn Through Real-World Scenarios: Analyze IC values from publicly available financial models. Avoid Pitfalls: Be aware of potential biases and limitations in IC calculation and interpretation. Think Creatively: Explore how to leverage IC insights to refine existing models and develop new ones. Go Beyond: Investigate the relationship between IC and other financial metrics like Sharpe ratio and turnover.
Conclusion: The Information Coefficient (IC) is more than a statistical measure—it's the compass guiding quantitative investors towards more informed decisions. By mastering its nuances, you unlock the art of evaluating predictive models, enhancing your ability to generate alpha and manage risk effectively.
Closing Message: Embrace the power of the Information Coefficient. Use it to critically assess your models, refine your strategies, and navigate the complexities of financial markets with increased confidence and success. The journey to mastering quantitative finance begins with understanding and effectively utilizing the IC.
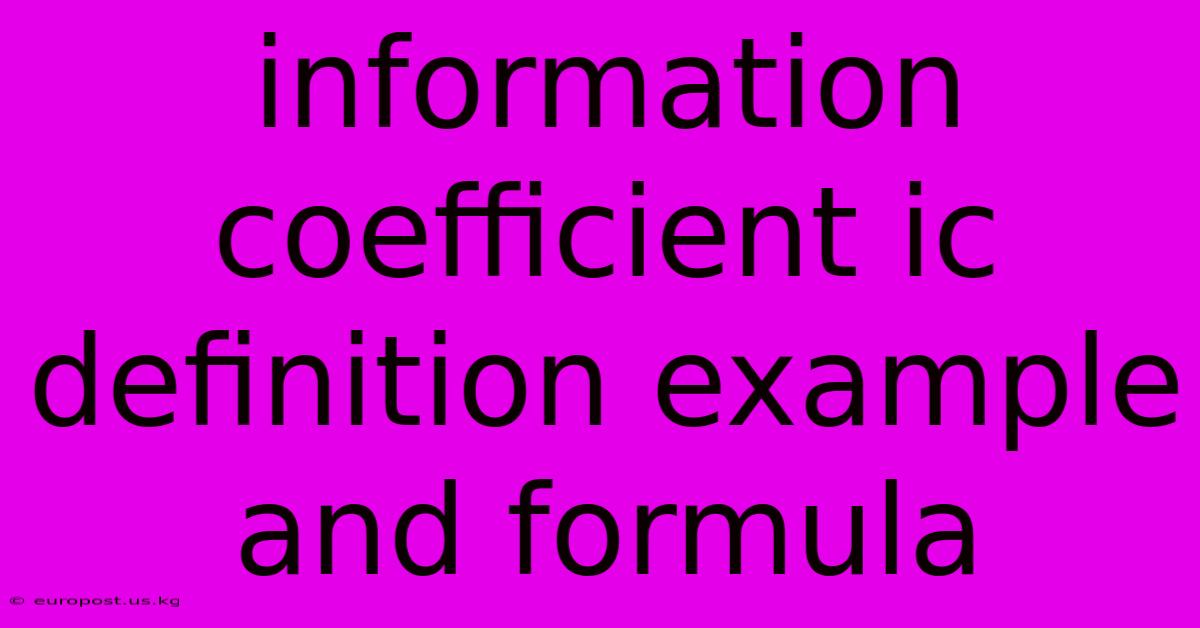
Thank you for taking the time to explore our website Information Coefficient Ic Definition Example And Formula. We hope you find the information useful. Feel free to contact us for any questions, and don’t forget to bookmark us for future visits!
We truly appreciate your visit to explore more about Information Coefficient Ic Definition Example And Formula. Let us know if you need further assistance. Be sure to bookmark this site and visit us again soon!
Featured Posts
-
Herfindahl Hirschman Index Hhi Definition Formula And Example
Jan 14, 2025
-
Independent Community Bankers Of America Icba Definition
Jan 14, 2025
-
Insider Trading Act Of 1988 Definition
Jan 14, 2025
-
Institutional Deposits Corporation Idc Definition
Jan 14, 2025
-
Intermediate Good Definition And Examples
Jan 14, 2025