Predictive Analytics Definition Model Types And Uses
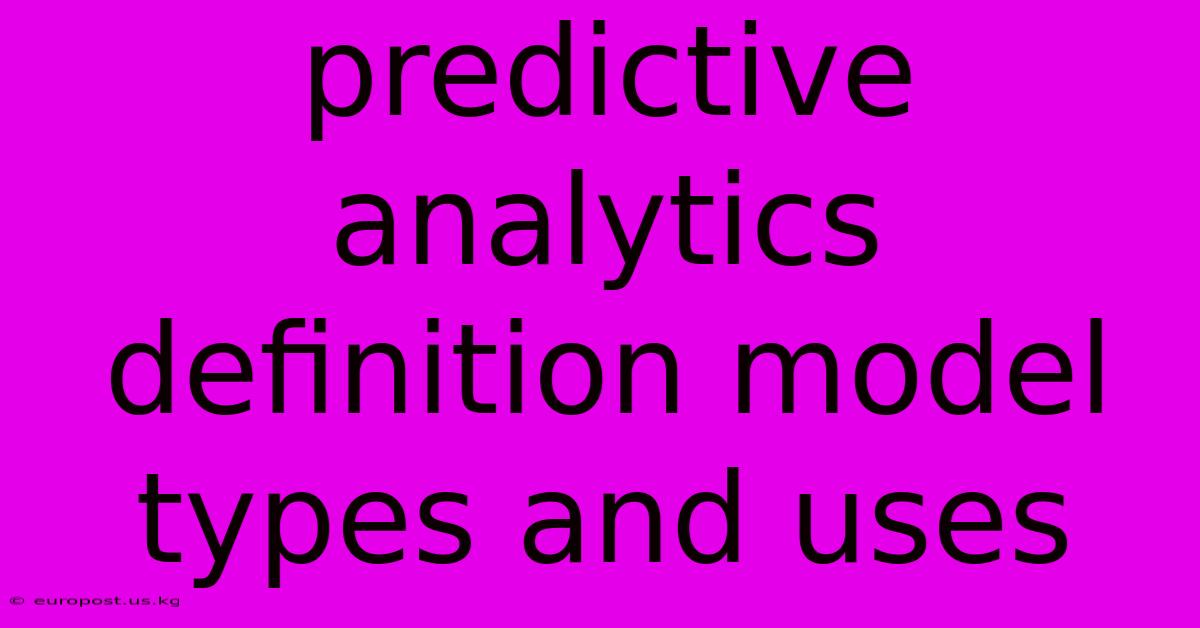
Discover more in-depth information on our site. Click the link below to dive deeper: Visit the Best Website meltwatermedia.ca. Make sure you don’t miss it!
Table of Contents
Unveiling the Secrets of Predictive Analytics: Exploring Its Pivotal Role in Decision-Making
Introduction: Dive into the transformative power of predictive analytics and its profound influence on data-driven decision-making. This detailed exploration offers expert insights and a fresh perspective that captivates business leaders, data scientists, and enthusiasts alike.
Hook: Imagine a world where you can anticipate customer behavior, predict market trends, and mitigate risks before they even materialize. This isn't science fiction; it's the reality enabled by predictive analytics. Beyond simply analyzing past data, predictive analytics leverages advanced algorithms to forecast future outcomes, empowering businesses to make proactive, informed decisions.
Editor’s Note: A groundbreaking new article on predictive analytics has just been released, uncovering its essential role in shaping strategic business decisions across diverse industries.
Why It Matters: In today's rapidly evolving business landscape, data is the new gold. However, raw data alone is insufficient. Predictive analytics transforms this raw data into actionable intelligence, providing a competitive edge by enabling businesses to:
- Proactively identify opportunities: Discover emerging market trends and unmet customer needs.
- Mitigate risks: Predict potential problems and take preventive measures, minimizing financial and reputational damage.
- Optimize resource allocation: Allocate resources more effectively based on anticipated demand and performance.
- Enhance customer experience: Personalize offerings and improve customer retention through predictive modeling of behavior.
- Improve operational efficiency: Streamline processes and reduce costs by anticipating bottlenecks and optimizing workflows.
Inside the Article
Breaking Down Predictive Analytics
Predictive analytics employs a variety of statistical techniques, machine learning algorithms, and data mining methods to analyze historical and current data and make predictions about future events. It moves beyond descriptive analytics (what happened) and diagnostic analytics (why it happened) to focus on what will happen.
Purpose and Core Functionality: The core purpose of predictive analytics is to forecast future outcomes based on past data patterns. This functionality relies heavily on sophisticated algorithms that identify relationships, trends, and patterns within datasets, often involving large volumes of data. These algorithms learn from the data and build models capable of making predictions.
Role in Decision-Making: Predictive analytics is not merely about generating predictions; it's about using those predictions to inform strategic decision-making. By providing insights into potential future scenarios, it allows businesses to proactively adapt their strategies, allocate resources more efficiently, and ultimately improve their bottom line.
Impact on Business Strategy: The impact of predictive analytics is far-reaching, influencing everything from marketing and sales to risk management and operations. It enables more data-driven decision-making, resulting in improved outcomes across the board.
Types of Predictive Analytics Models:
Several types of models are commonly used in predictive analytics, each with its strengths and weaknesses:
-
Regression Models: These models predict a continuous outcome variable (e.g., sales revenue, stock price) based on one or more predictor variables. Linear regression, logistic regression, and polynomial regression are common examples. They are relatively simple to interpret but may not capture complex relationships.
-
Classification Models: These models predict a categorical outcome variable (e.g., customer churn, loan default) by assigning data points to predefined classes. Decision trees, support vector machines (SVMs), and naive Bayes classifiers are frequently employed. They are useful for classifying data into distinct groups.
-
Clustering Models: These models group similar data points together without pre-defined classes. K-means clustering and hierarchical clustering are popular methods. They are useful for identifying hidden patterns and segments within data.
-
Time Series Models: These models predict future values of a variable based on its historical values over time. ARIMA models and exponential smoothing are commonly used. They are essential for forecasting trends in data that changes over time.
-
Neural Networks: These models are inspired by the structure and function of the human brain. They can handle complex relationships and large datasets but can be difficult to interpret. Deep learning, a subset of neural networks, has gained immense popularity due to its ability to handle massive datasets and extract intricate patterns.
Exploring the Depth of Predictive Analytics
Opening Statement: What if you could anticipate customer needs before they even articulate them? Predictive analytics empowers businesses to do just that. It moves beyond reacting to events to proactively shaping the future.
Core Components: The core components of predictive analytics include data collection, data preprocessing, model selection, model training, model evaluation, and deployment. Each stage is crucial for building accurate and reliable predictive models.
In-Depth Analysis: Real-world examples demonstrate the power of predictive analytics. For instance, in healthcare, it can predict patient readmission rates, enabling proactive interventions to improve patient outcomes. In finance, it can assess credit risk, reducing loan defaults. In marketing, it can personalize recommendations, boosting sales conversions.
Interconnections: Predictive analytics often works in conjunction with other data analysis techniques, such as descriptive and diagnostic analytics. The insights gained from these complementary approaches provide a more comprehensive understanding of the data and lead to more informed decisions.
FAQ: Decoding Predictive Analytics
What does predictive analytics do? It uses historical data to predict future outcomes, providing businesses with actionable insights for better decision-making.
How accurate are predictive analytics models? Accuracy depends on several factors, including data quality, model selection, and the complexity of the phenomenon being predicted. Model evaluation metrics provide insights into the reliability of predictions.
What are the limitations of predictive analytics? Limitations include data bias, overfitting (models that perform well on training data but poorly on new data), and the inability to predict unforeseen events.
Is predictive analytics only for large businesses? While large businesses often have the resources to implement sophisticated predictive analytics systems, smaller businesses can also benefit from simpler models and readily available tools.
What are the ethical considerations of predictive analytics? Ethical concerns include data privacy, bias in algorithms, and the potential for discriminatory outcomes. Responsible use of predictive analytics requires careful consideration of these ethical implications.
Practical Tips to Master Predictive Analytics
-
Start with the Basics: Begin with a clear understanding of the business problem you are trying to solve. Define your objectives and identify the key variables.
-
Data Quality is Paramount: Ensure your data is accurate, complete, and relevant. Data cleaning and preprocessing are essential steps.
-
Choose the Right Model: Select a model appropriate for your data and the type of prediction you need. Experiment with different models and compare their performance.
-
Validate Your Model: Rigorously test your model using appropriate evaluation metrics to assess its accuracy and reliability.
-
Iterate and Improve: Predictive analytics is an iterative process. Continuously monitor your models, refine them based on new data, and adapt them to changing circumstances.
Conclusion: Predictive analytics is more than a technological advancement—it's a transformative force reshaping business decision-making. By mastering its nuances and ethically leveraging its power, businesses can unlock new opportunities, mitigate risks, and achieve sustainable growth in an increasingly data-driven world.
Closing Message: Embrace the power of predictive analytics and unlock a future where data-driven insights guide your every strategic move. The journey may require expertise and careful planning, but the rewards are well worth the effort. The future of effective business strategy is predictive.
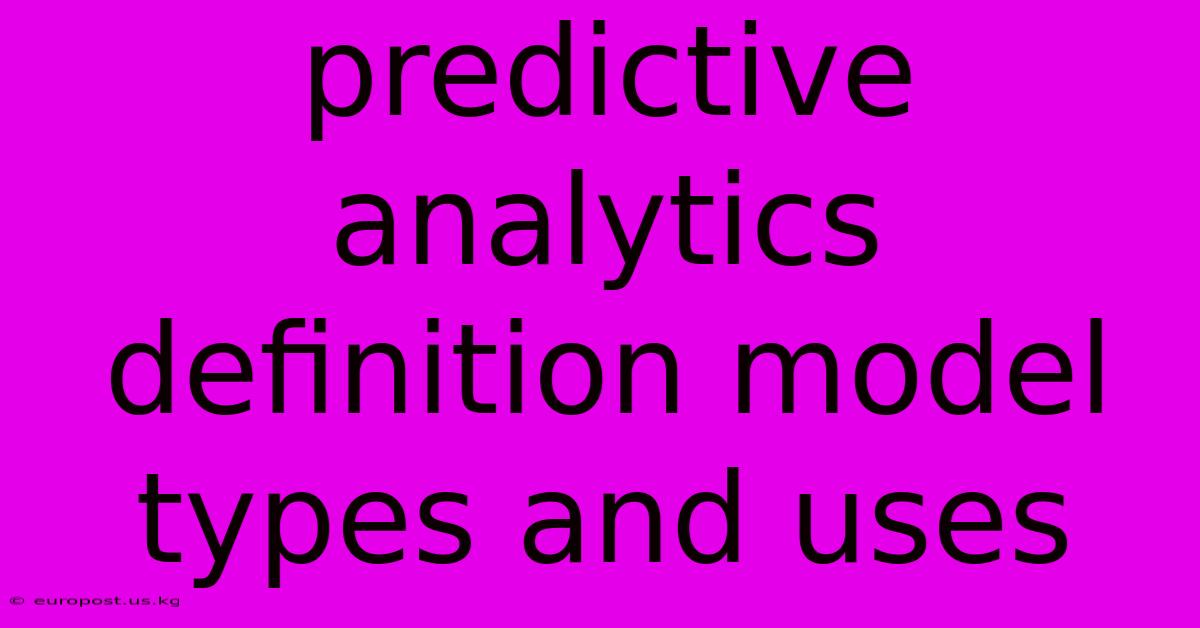
Thank you for taking the time to explore our website Predictive Analytics Definition Model Types And Uses. We hope you find the information useful. Feel free to contact us for any questions, and don’t forget to bookmark us for future visits!
We truly appreciate your visit to explore more about Predictive Analytics Definition Model Types And Uses. Let us know if you need further assistance. Be sure to bookmark this site and visit us again soon!
Featured Posts
-
What Are The Qualifications For A Walmart Credit Card
Jan 21, 2025
-
What Is A Credit Week For Pa Unemployment
Jan 21, 2025
-
Variable Annuitization Definition
Jan 21, 2025
-
Predicting Ohio State Vs Notre Dame 2025
Jan 21, 2025
-
Bidens Last Minute Sibling Pardons
Jan 21, 2025