Residual Standard Deviation Definition Formula And Examples
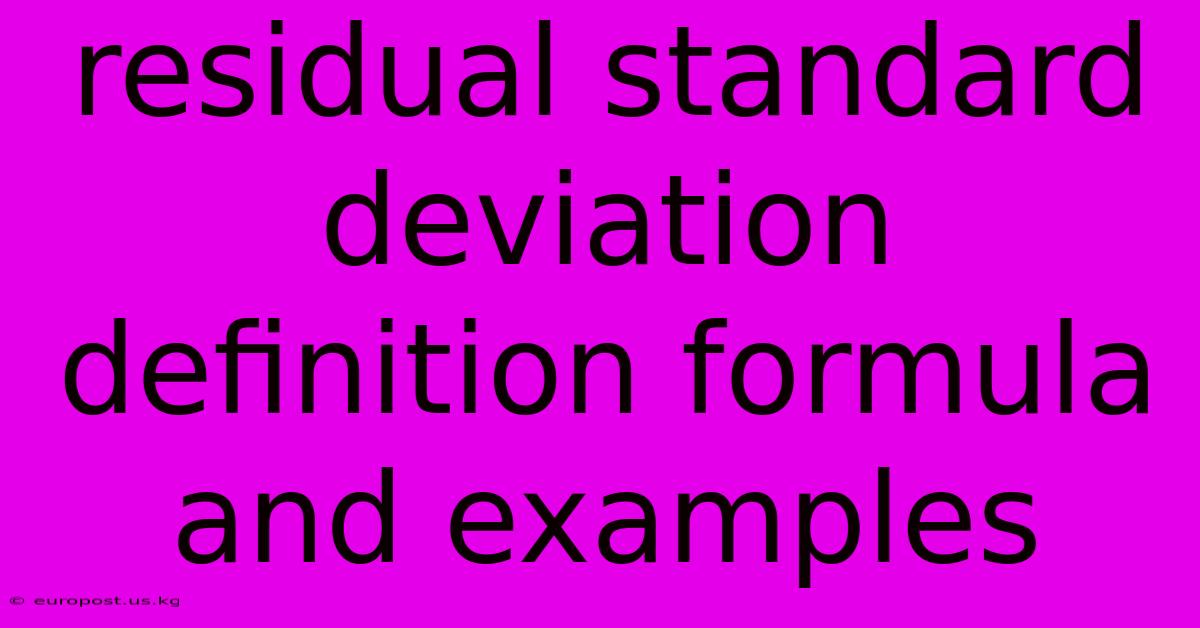
Discover more in-depth information on our site. Click the link below to dive deeper: Visit the Best Website meltwatermedia.ca. Make sure you don’t miss it!
Table of Contents
Unveiling the Secrets of Residual Standard Deviation: Exploring Its Pivotal Role in Regression Analysis
Introduction: Dive into the transformative power of residual standard deviation and its profound influence on regression analysis and understanding model fit. This detailed exploration offers expert insights and a fresh perspective that captivates statisticians, data scientists, and students alike.
Hook: Imagine if you could quantify the unexplained variability in your regression model—the unpredictable noise that remains even after accounting for the predictor variables. That's the power of residual standard deviation. It's not just a number; it's a crucial metric revealing the accuracy and reliability of your model's predictions.
Editor’s Note: A groundbreaking new article on residual standard deviation has just been released, uncovering its essential role in assessing the goodness of fit in regression models.
Why It Matters: Residual standard deviation (RSD) is a cornerstone of regression analysis. It measures the spread of the residuals—the differences between observed and predicted values. A smaller RSD indicates a better-fitting model, suggesting that the model explains a larger portion of the variability in the dependent variable. Understanding RSD is essential for interpreting model performance, making accurate predictions, and drawing reliable conclusions from your data.
Inside the Article
Breaking Down Residual Standard Deviation
Purpose and Core Functionality: The primary purpose of RSD is to quantify the unexplained variation in a regression model. It essentially represents the average distance of the data points from the regression line. A smaller RSD implies that the data points cluster tightly around the regression line, indicating a strong fit. Conversely, a larger RSD suggests a weaker fit, with data points scattered further from the line.
Role in Sentence Structure: (Note: This section is a bit of a misnomer given the context of statistical analysis. We will instead discuss the role of RSD in the overall structure of regression analysis.) RSD plays a critical role in the overall structure of regression analysis by providing a measure of the model's accuracy. It helps to complete the picture of model performance alongside other metrics like R-squared and the coefficient of determination. It's an integral part of assessing the overall validity and usefulness of the regression model.
Impact on Interpretation and Inference: RSD significantly impacts the interpretation and inferences drawn from a regression model. A low RSD suggests high predictive power, implying that the model is reliable for forecasting. Conversely, a high RSD suggests that the model's predictions are less reliable due to significant unexplained variability. This understanding is crucial for making informed decisions based on the model's outputs.
Formula and Calculation:
The residual standard deviation is calculated as the square root of the Mean Squared Error (MSE). The formula is:
RSD = √(MSE) = √[∑(yi - ŷi)² / (n - k)]
Where:
- yi represents the observed value of the dependent variable.
- ŷi represents the predicted value of the dependent variable from the regression model.
- n represents the number of observations.
- k represents the number of predictor variables (including the intercept).
- ∑ represents the sum of the squared differences between observed and predicted values.
The denominator (n-k) represents the degrees of freedom, accounting for the parameters estimated in the model. Using degrees of freedom ensures unbiased estimation of the variance.
Exploring the Depth of Residual Standard Deviation
Opening Statement: What if there were a single metric capable of summarizing the remaining uncertainty in your regression model? That's the essence of residual standard deviation. It not only quantifies the model's error but also provides insights into the reliability of its predictions.
Core Components: The core of RSD lies in its ability to capture the average deviation of the data points from the regression line. It's derived directly from the residuals, which are the differences between the actual and predicted values. These residuals represent the unexplained portion of the dependent variable's variability. Understanding the distribution of residuals is also crucial; ideally, they should be normally distributed with a mean of zero.
In-Depth Analysis: Let's consider a simple linear regression model predicting house prices (dependent variable) based on house size (independent variable). If the RSD is low, it suggests that the model accurately predicts house prices based on size, with minimal unexplained variability. A high RSD, on the other hand, indicates that other factors significantly influence house prices beyond just size, leading to less accurate predictions.
Interconnections: RSD is closely related to other key statistical measures in regression analysis. For example, it's linked to the R-squared value, which measures the proportion of variance in the dependent variable explained by the model. A low RSD typically corresponds to a high R-squared, indicating a good model fit. It is also related to the standard error of the regression, which essentially represents the standard deviation of the residuals.
FAQ: Decoding Residual Standard Deviation
What does residual standard deviation do? It measures the average amount of error in a regression model's predictions. It essentially quantifies the scatter of data points around the regression line.
How does it influence interpretation? A low RSD suggests a well-fitting model with accurate predictions, while a high RSD indicates a poor fit and less reliable predictions.
Is it always relevant? Yes, RSD is relevant in any regression analysis where the goal is to assess the model's predictive accuracy and understand the unexplained variability in the data.
What happens when RSD is high? A high RSD implies that the model doesn't explain a significant portion of the dependent variable's variability, suggesting the need to explore additional predictor variables or consider alternative modeling approaches.
Is RSD affected by outliers? Yes, outliers can significantly inflate the RSD as they contribute disproportionately to the sum of squared errors. Outlier detection and handling are crucial for obtaining a reliable RSD.
Practical Tips to Master Residual Standard Deviation
Start with the Basics: Understand the concept of residuals and how they represent the difference between observed and predicted values.
Step-by-Step Application: Learn how to calculate the MSE and then take the square root to obtain the RSD. Utilize statistical software like R, Python (with libraries like Statsmodels or Scikit-learn), or SPSS to automate this calculation.
Learn Through Real-World Scenarios: Analyze real datasets and interpret the RSD in the context of the specific problem. Consider examples from various fields like finance, economics, or engineering.
Avoid Pitfalls: Be aware of the impact of outliers and consider appropriate data cleaning techniques. Understand that a low RSD doesn't automatically guarantee a good model; it's just one piece of the puzzle.
Think Creatively: Explore how RSD can help you compare different regression models and select the one that best fits your data.
Conclusion: Residual standard deviation is more than a statistical measure—it's a critical tool for evaluating the accuracy and reliability of regression models. By mastering its nuances and understanding its relationship to other statistical measures, you gain invaluable insights into the strength of your model and the confidence you can place in its predictions.
Closing Message: Embrace the power of residual standard deviation to enhance your understanding of regression analysis. By effectively utilizing this metric, you can move beyond simple model fitting and gain deeper insights into the underlying data patterns, leading to more accurate predictions and informed decisions. Remember to always consider RSD in conjunction with other diagnostic tools to gain a comprehensive understanding of your regression model's performance.
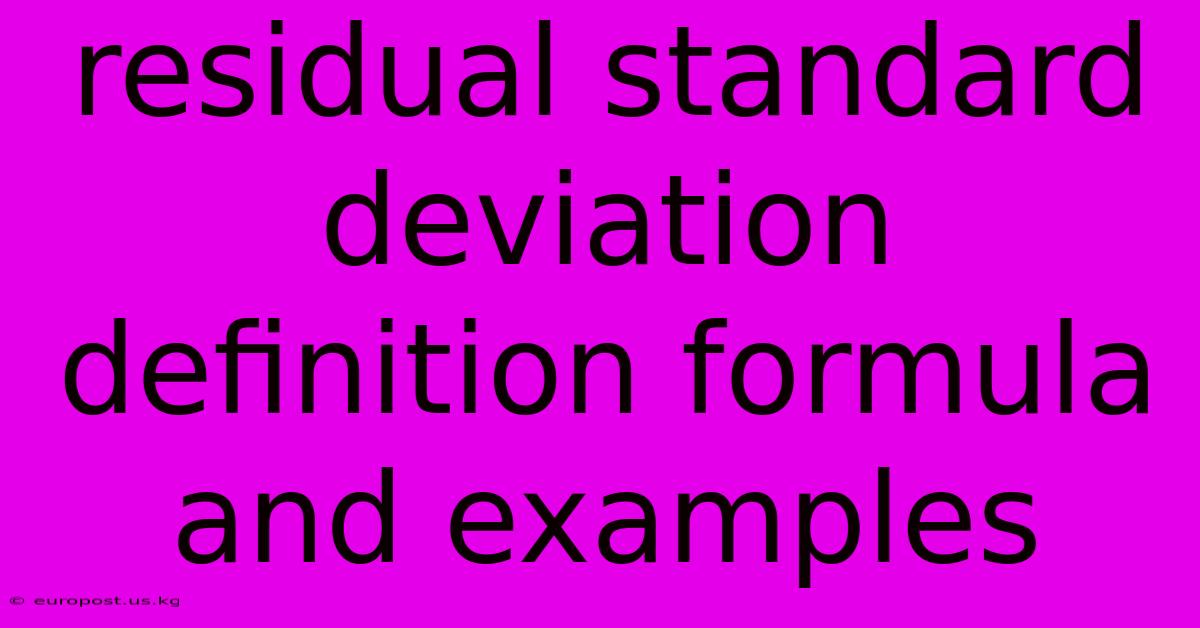
Thank you for taking the time to explore our website Residual Standard Deviation Definition Formula And Examples. We hope you find the information useful. Feel free to contact us for any questions, and don’t forget to bookmark us for future visits!
We truly appreciate your visit to explore more about Residual Standard Deviation Definition Formula And Examples. Let us know if you need further assistance. Be sure to bookmark this site and visit us again soon!
Featured Posts
-
Mortgage Accelerator Definition
Jan 16, 2025
-
What Is Ssr Stocks
Jan 16, 2025
-
What Is An Sma In Stocks
Jan 16, 2025
-
How To Sell Stocks On Marketwatch
Jan 16, 2025
-
Destaques Do Cruzeiro Matheus E Dudu
Jan 16, 2025