Sample Selection Bias Definition Examples And How To Avoid
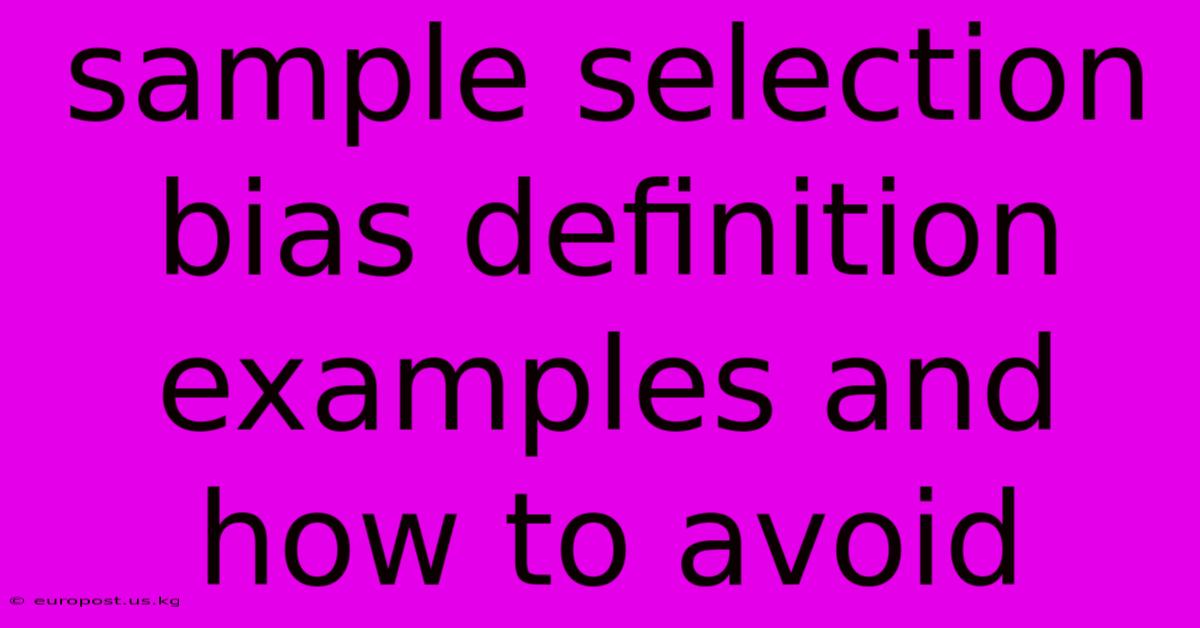
Discover more in-depth information on our site. Click the link below to dive deeper: Visit the Best Website meltwatermedia.ca. Make sure you don’t miss it!
Table of Contents
Unveiling the Secrets of Sample Selection Bias: Exploring Its Pivotal Role in Research
Introduction: Dive into the transformative power of understanding sample selection bias and its profound influence on the validity of research findings. This detailed exploration offers expert insights and a fresh perspective that captivates researchers, students, and anyone interested in data analysis.
Hook: Imagine investing significant time and resources in a research project, only to discover your conclusions are skewed because of a flaw in how you selected your participants. This is the insidious impact of sample selection bias. It's not a simple oversight; it's a systematic error that can invalidate entire studies, leading to inaccurate conclusions and potentially harmful consequences.
Editor’s Note: A groundbreaking new article on sample selection bias has just been released, uncovering its essential role in shaping the reliability of research findings.
Why It Matters: Sample selection bias is the cornerstone of flawed research. It influences the generalizability of findings, meaning the conclusions drawn from a biased sample may not accurately reflect the larger population being studied. This deep dive reveals its critical role in undermining statistical analysis, leading to incorrect interpretations and potentially misleading policy decisions. Understanding and mitigating selection bias is crucial for producing credible and impactful research across all fields.
Inside the Article
Breaking Down Sample Selection Bias
Purpose and Core Functionality: Sample selection bias occurs when the method of selecting participants for a study results in a sample that is not representative of the population from which it is drawn. This non-representativeness introduces systematic error, leading to inaccurate estimations and potentially spurious correlations. The bias doesn't arise from random chance; it's a consequence of the selection process itself.
Role in Study Design: The design of the sampling methodology is paramount. If researchers use convenience sampling (selecting easily accessible participants) or volunteer sampling (relying on individuals who choose to participate), the risk of bias dramatically increases. These methods often attract specific subgroups, skewing the sample and undermining the study's validity. For example, a study on internet usage conducted solely among university students would likely overestimate internet access and proficiency in the general population.
Impact on Results and Interpretation: The consequences of sample selection bias are far-reaching. It can lead to:
- Overestimation or underestimation of effects: A biased sample might exaggerate or diminish the true effect size of a treatment or intervention.
- Spurious correlations: Relationships between variables might appear to exist when they don't, solely due to the skewed representation of the population.
- Incorrect generalizations: Conclusions drawn from a biased sample cannot be reliably generalized to the broader population.
- Erroneous policy decisions: Research findings influenced by selection bias can lead to ineffective or even harmful policies based on inaccurate data.
Exploring the Depth of Sample Selection Bias
Opening Statement: What if the foundation of your research was inherently flawed? That's the reality of unchecked sample selection bias. It not only distorts the picture but also compromises the integrity of the entire research process.
Core Components: Sample selection bias manifests in various forms:
- Self-selection bias: This occurs when participants choose whether or not to participate, leading to a sample that differs systematically from the population. For example, a study on the effectiveness of a weight-loss program might attract highly motivated individuals, leading to an overestimation of its effectiveness.
- Convenience sampling bias: This arises when researchers select participants based on ease of access, such as using students or readily available employees. This can result in samples that are not representative of the larger population.
- Exclusion bias: This happens when specific groups are systematically excluded from the study, leading to an incomplete picture. For instance, excluding older adults from a study on technology adoption would create a biased sample.
- Attrition bias: This occurs when participants drop out of a longitudinal study, and those who remain differ systematically from those who dropped out. This can lead to biased conclusions about the long-term effects of a treatment or intervention.
- Healthy user bias: This occurs in observational studies where those choosing to participate (e.g., in a health program) might be inherently healthier or more proactive than those who don't, making it difficult to isolate the effect of the program.
In-Depth Analysis: Consider a study examining the link between coffee consumption and heart disease. If the sample predominantly consists of individuals from a specific socioeconomic group with different dietary habits and access to healthcare, the findings might not reflect the true relationship between coffee and heart health in the general population.
Interconnections: Sample selection bias often interacts with other biases, such as reporting bias (selective reporting of results) and confirmation bias (interpreting data to confirm pre-existing beliefs), exacerbating the problem and further undermining the research's credibility.
FAQ: Decoding Sample Selection Bias
What does sample selection bias do? It introduces systematic error into research, leading to inaccurate estimates and misleading conclusions.
How does it influence meaning? By skewing the sample, it distorts the representation of the population, altering the interpretation of the results and limiting their generalizability.
Is it always relevant? Yes, it's a potential problem in virtually any type of research involving sampling, especially observational studies.
What happens when sample selection bias is ignored? Invalid conclusions, wasted resources, and potentially harmful policy decisions can result.
How can we identify sample selection bias? By carefully examining the sampling methods, comparing the characteristics of the sample to the population, and considering potential sources of bias in the selection process.
Practical Tips to Master Avoiding Sample Selection Bias
Start with the Basics: Define your target population precisely. Clearly specify the characteristics of the individuals you want to study.
Step-by-Step Application: Employ rigorous sampling techniques, such as random sampling (simple, stratified, cluster), to ensure a representative sample. Consider using probability sampling methods to increase the chances of a representative sample.
Learn Through Real-World Scenarios: Analyze past studies to understand how selection bias has impacted their findings and learn from the mistakes made.
Avoid Pitfalls: Be cautious of convenience sampling and self-selection bias. Actively seek to minimize attrition in longitudinal studies.
Think Creatively: Employ strategies like weighting the data to adjust for known biases, or using statistical techniques to account for selection bias in the analysis.
Go Beyond: Conduct power analysis to determine the appropriate sample size needed to detect meaningful effects and minimize the influence of random error. Clearly report your sampling methods and any potential limitations in your study.
Conclusion: Sample selection bias is more than a methodological hiccup; it's a fundamental threat to the validity and reliability of research findings. By mastering the techniques to avoid it, you unlock the art of conducting robust research, enhancing the impact and credibility of your work, and contributing to a more informed understanding of the world.
Closing Message: Embrace the power of rigorous sampling methods and meticulous study design. By actively addressing sample selection bias, you pave the way for impactful and trustworthy research, making a meaningful contribution to your field and beyond. Remember, the pursuit of accurate knowledge demands attention to detail and a commitment to eliminating bias at every stage of the research process.
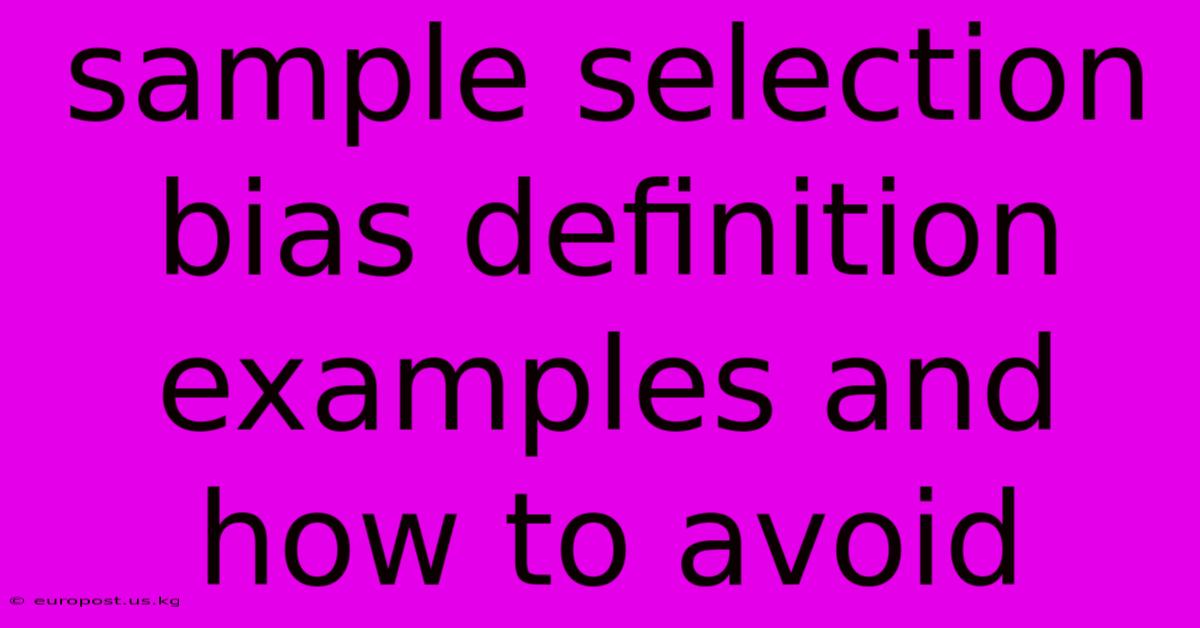
Thank you for taking the time to explore our website Sample Selection Bias Definition Examples And How To Avoid. We hope you find the information useful. Feel free to contact us for any questions, and don’t forget to bookmark us for future visits!
We truly appreciate your visit to explore more about Sample Selection Bias Definition Examples And How To Avoid. Let us know if you need further assistance. Be sure to bookmark this site and visit us again soon!
Featured Posts
-
Sec Form 485a24f Definition
Jan 17, 2025
-
Which Results Are More Likely For Someone Without Personal Finance Skills
Jan 17, 2025
-
David Lynch Dies A Filmmakers Passing
Jan 17, 2025
-
Acceptor Definition
Jan 17, 2025
-
What Is Actuarial Science Definition And Examples Of Application
Jan 17, 2025