Symmetrical Distribution Defined What It Tells You And Examples
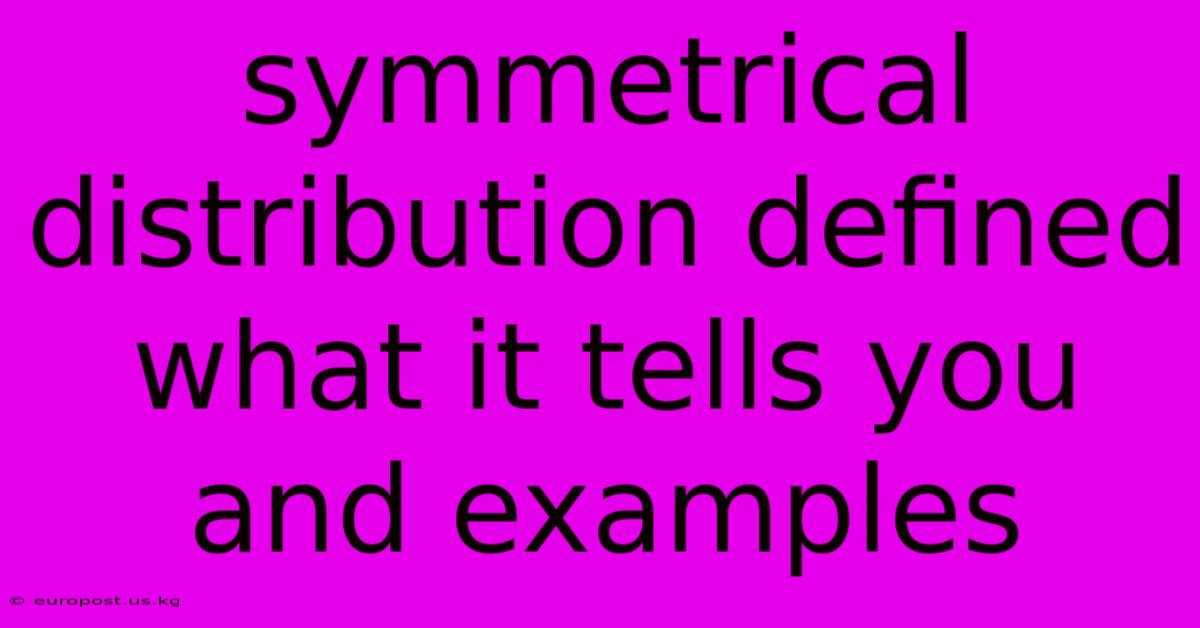
Discover more in-depth information on our site. Click the link below to dive deeper: Visit the Best Website meltwatermedia.ca. Make sure you don’t miss it!
Table of Contents
Unveiling the Secrets of Symmetrical Distribution: Exploring Its Pivotal Role in Data Analysis
Introduction: Dive into the transformative power of symmetrical distribution and its profound influence on data analysis and interpretation. This detailed exploration offers expert insights and a fresh perspective that captivates statisticians, data scientists, and anyone interested in understanding data more effectively.
Hook: Imagine if you could instantly grasp the central tendency and variability of a dataset with just a glance. Symmetrical distribution makes this possible. Beyond being just a statistical concept, it's the invisible force that simplifies complex data, revealing patterns and insights that would otherwise be hidden.
Editor’s Note: A groundbreaking new article on symmetrical distribution has just been released, uncovering its essential role in shaping data-driven decision-making.
Why It Matters: Symmetrical distribution is the cornerstone of many statistical analyses. Understanding its characteristics allows for efficient data summarization, accurate hypothesis testing, and reliable predictions. This deep dive reveals its critical role in identifying normality, simplifying calculations, and facilitating meaningful interpretations—unlocking strategies for success in various fields from finance to healthcare.
Inside the Article
Breaking Down Symmetrical Distribution
Purpose and Core Functionality: A symmetrical distribution, also known as a symmetrical probability distribution, is a type of probability distribution where the probability density function (PDF) is symmetric around its mean. This means that the data points are evenly distributed on both sides of the mean. The visual representation of a symmetrical distribution is often a bell-shaped curve, although not all symmetrical distributions are bell-shaped. The key characteristic is the perfect mirror image around the central point.
Role in Data Summarization: In symmetrical distributions, the mean, median, and mode are all equal. This simplifies data summarization significantly. Instead of needing multiple measures of central tendency, a single value (the mean) provides a complete picture of the "center" of the data. This efficiency is invaluable when dealing with large datasets.
Impact on Inferential Statistics: Symmetrical distributions, particularly the normal distribution, are the foundation for many inferential statistical tests. These tests rely on the assumptions of normality to ensure the validity of their results. Understanding the symmetry of your data helps determine the appropriateness of these widely-used statistical methods.
Exploring the Depth of Symmetrical Distribution
Opening Statement: What if there were a statistical concept so integral it simplifies complex data analysis and improves the reliability of your conclusions? That’s symmetrical distribution. It shapes not only the interpretation of data but also the choice of statistical methods used for analysis.
Core Components: The essence of symmetrical distribution lies in its balanced nature. The data points spread equally on both sides of the mean, creating a visually appealing and mathematically convenient structure. This balance directly impacts how we calculate and interpret statistical measures such as standard deviation and variance.
In-Depth Analysis: Let's examine a real-world example. Consider the heights of adult women in a specific population. If we plot the data, we might observe a roughly symmetrical distribution, with the mean height representing the most frequent height. The distribution would show a gradual decrease in frequency as we move away from the mean, both towards shorter and taller heights. This symmetrical nature simplifies the calculation of the average height and allows us to make inferences about the population's height distribution.
Interconnections: The relationship between symmetrical distribution and the normal distribution is crucial. The normal distribution is a specific type of symmetrical distribution, and it's arguably the most important distribution in statistics. Many statistical tests and models assume that data follows a normal distribution, making understanding its symmetrical nature paramount.
FAQ: Decoding Symmetrical Distribution
What does symmetrical distribution do? It simplifies data analysis by providing a straightforward way to describe the center and spread of the data. The equality of mean, median, and mode provides a robust summary.
How does it influence inferences? It allows us to use parametric statistical tests, which are generally more powerful and efficient than non-parametric tests, provided the data truly fits a symmetrical model.
Is it always relevant? While many real-world datasets approximate symmetrical distributions, it's important to note that not all data is symmetrically distributed. Skewed distributions, for example, require different analytical approaches.
What happens when symmetrical distribution is assumed incorrectly? Using methods that assume symmetry when the data is skewed can lead to inaccurate conclusions and biased results. Appropriate tests for normality should always be conducted.
Is symmetrical distribution the same across all fields? The underlying principle remains consistent across disciplines, but the specific shape and context of the symmetrical distribution might vary depending on the data being analyzed.
Practical Tips to Master Symmetrical Distribution
Start with the Basics: Visualize your data using histograms or box plots to get a sense of its distribution. Look for visual symmetry around the central point.
Step-by-Step Application: Calculate the mean, median, and mode. If these values are approximately equal, it suggests a symmetrical distribution. Check for normality using statistical tests like the Shapiro-Wilk test or Kolmogorov-Smirnov test.
Learn Through Real-World Scenarios: Analyze datasets from different fields, noting the presence or absence of symmetry and its implications for analysis.
Avoid Pitfalls: Don't automatically assume symmetry. Always visually inspect and statistically test for normality before applying methods that rely on this assumption.
Think Creatively: Consider data transformations (e.g., logarithmic transformations) to make skewed data more symmetrical if appropriate for the analysis.
Go Beyond: Explore different types of symmetrical distributions beyond the normal distribution, such as the uniform distribution.
Examples of Symmetrical Distributions
- Heights of adult males: The distribution of heights within a population tends to be approximately symmetrical, clustering around the average height.
- Test scores: In a well-designed test, scores might be symmetrically distributed around the average score.
- Measurement errors: Random errors in measurement often follow a symmetrical distribution, with positive and negative deviations equally likely.
- Simulated data: In simulations, symmetrical distributions are often used as a basis for creating random numbers or representing certain phenomena.
Conclusion: Symmetrical distribution is more than a statistical tool—it’s the key to unlocking accurate and efficient data analysis. By mastering its nuances, you unlock the art of insightful data interpretation, enhancing the reliability of your conclusions in every field.
Closing Message: Embrace the power of understanding symmetrical distributions. By recognizing its presence and understanding its implications, you equip yourself to make more informed decisions based on data-driven insights. This knowledge empowers you to move beyond simple descriptions to a deeper understanding of the patterns and truths hidden within your data.
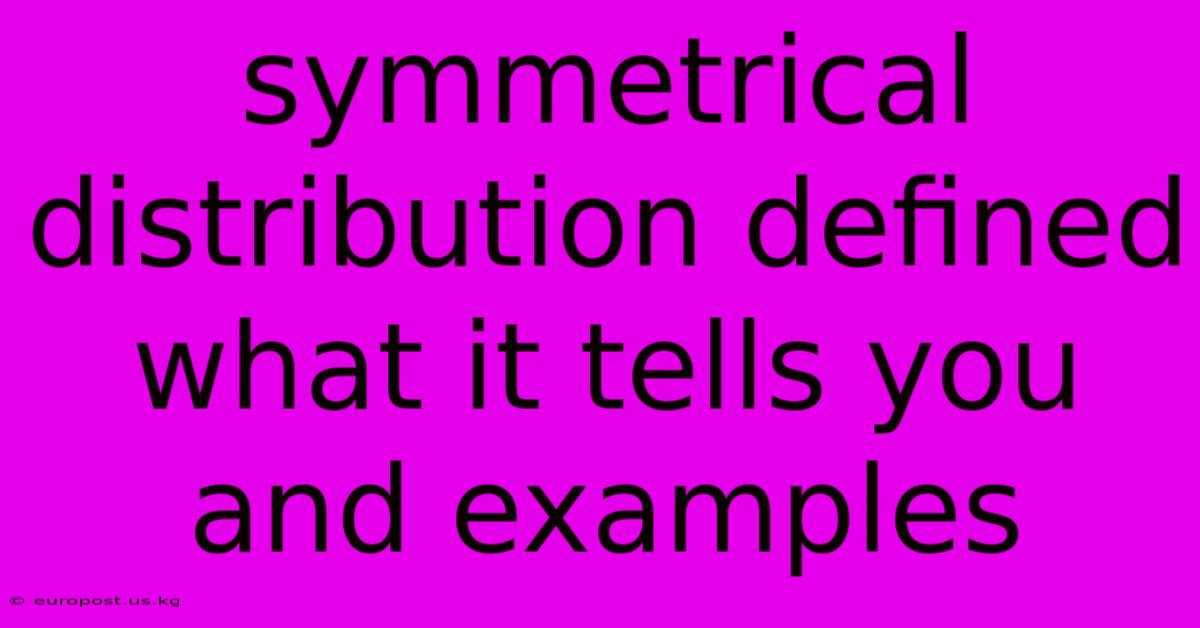
Thank you for taking the time to explore our website Symmetrical Distribution Defined What It Tells You And Examples. We hope you find the information useful. Feel free to contact us for any questions, and don’t forget to bookmark us for future visits!
We truly appreciate your visit to explore more about Symmetrical Distribution Defined What It Tells You And Examples. Let us know if you need further assistance. Be sure to bookmark this site and visit us again soon!
Featured Posts
-
Squeezes Definition How They Work Types And Examples
Jan 19, 2025
-
Tangible Book Value Per Share Tbvps Definition And Formula
Jan 19, 2025
-
State Administrator Definition
Jan 19, 2025
-
Step Up Bond Definition How It Works Pros Cons Example
Jan 19, 2025
-
Trump Trumps Pre Return Coin
Jan 19, 2025