Type 1 Error Definition False Positives And Examples
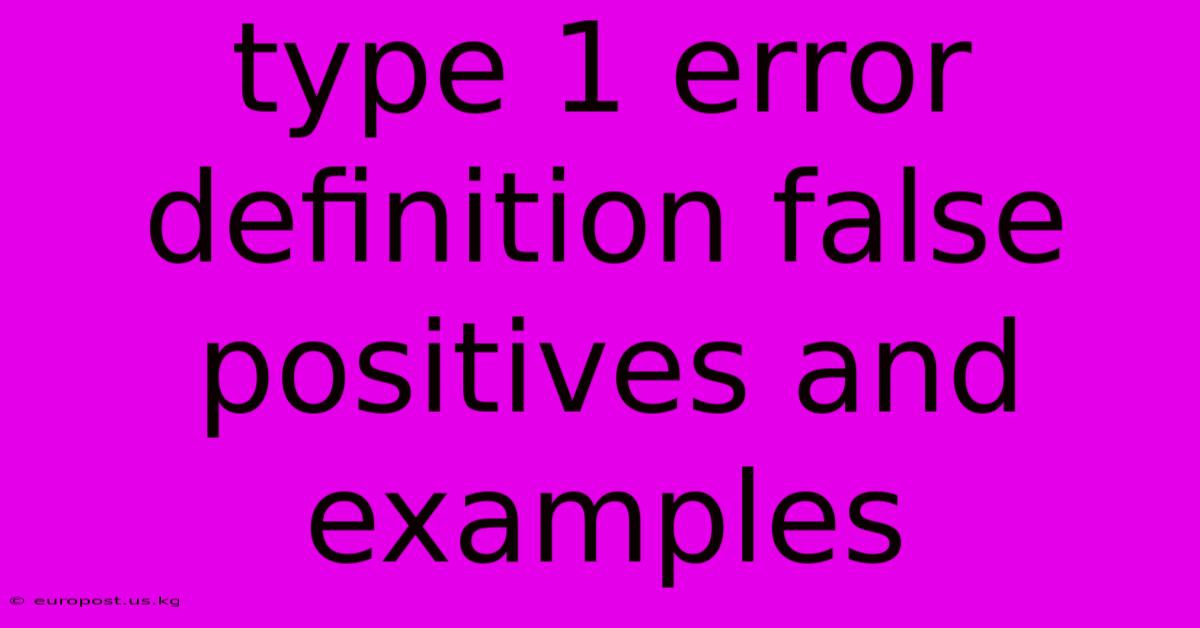
Discover more in-depth information on our site. Click the link below to dive deeper: Visit the Best Website meltwatermedia.ca. Make sure you don’t miss it!
Table of Contents
Unveiling the Secrets of Type I Error: Exploring Its Pivotal Role in Statistical Decision-Making
Introduction: Dive into the transformative power of understanding Type I error and its profound influence on statistical analysis and decision-making. This detailed exploration offers expert insights and a fresh perspective that captivates professionals and enthusiasts alike.
Hook: Imagine making a critical decision based on flawed data, leading to significant consequences. This scenario highlights the crucial importance of understanding Type I error, a concept that lies at the heart of statistical hypothesis testing. A Type I error, also known as a false positive, is more than just a statistical anomaly; it's a potential pitfall that can lead to incorrect conclusions with real-world ramifications.
Editor’s Note: A groundbreaking new article on Type I Error has just been released, uncovering its essential role in shaping reliable statistical inferences.
Why It Matters: Type I error is the cornerstone of sound statistical practice. It influences how we interpret data, design experiments, and ultimately, make informed decisions. This deep dive reveals its critical role in various fields, from medicine and finance to engineering and social sciences—unlocking strategies for minimizing its impact and increasing the reliability of our conclusions.
Inside the Article
Breaking Down Type I Error
Purpose and Core Functionality: A Type I error occurs when we reject a true null hypothesis. In simpler terms, it's concluding something is true when it's actually false. The null hypothesis (H₀) is a statement of no effect or no difference. Rejecting it implies finding evidence for an alternative hypothesis (H₁). A Type I error means we've wrongly found evidence for this alternative.
Role in Hypothesis Testing: Hypothesis testing involves comparing observed data to what we'd expect under the null hypothesis. We use statistical tests to calculate a p-value, representing the probability of observing our data (or more extreme data) if the null hypothesis were true. If the p-value is below a pre-determined significance level (alpha, often 0.05), we reject the null hypothesis. If we reject a true null hypothesis, we've committed a Type I error.
Impact on Decision-Making: The consequences of a Type I error can be substantial. In medical research, it might lead to approving a drug that's ineffective or even harmful. In finance, it could result in investing in a failing company. The severity depends heavily on the context and the cost associated with making an incorrect decision.
Examples of Type I Errors Across Disciplines
Medicine: Imagine a new drug trial designed to reduce blood pressure. The null hypothesis is that the drug has no effect. If the trial concludes the drug is effective (rejecting the null hypothesis) when, in reality, it has no effect, that's a Type I error. This could lead to widespread adoption of an ineffective medication, potentially wasting resources and potentially harming patients.
Finance: A stock screening algorithm might signal a strong buy for a particular stock based on its historical performance. If this signal leads to investment, and the stock subsequently underperforms significantly, a Type I error has occurred. The algorithm falsely identified a profitable opportunity.
Quality Control: In manufacturing, a quality control test might indicate a batch of products is defective when, in fact, it meets the quality standards. This could lead to discarding perfectly good products, resulting in financial losses and reduced production efficiency.
Social Sciences: A study investigating the relationship between social media usage and depression might find a significant correlation where none exists. This Type I error could lead to inaccurate conclusions about the causes of depression and potentially influence policy decisions based on flawed data.
Exploring the Depth of Type I Error
Core Components: Significance Level (Alpha): The significance level (alpha) is the probability of committing a Type I error. It's a pre-determined threshold, often set at 0.05 (5%). This means there's a 5% chance of rejecting a true null hypothesis even when using a statistically sound test.
Power of a Test: The power of a statistical test is the probability of correctly rejecting a false null hypothesis (avoiding a Type II error). Higher power means a lower chance of a Type II error, but it doesn't directly address the risk of a Type I error. A highly powerful test can still produce a Type I error if the null hypothesis is true.
Multiple Comparisons Problem: When performing multiple statistical tests simultaneously, the probability of making at least one Type I error increases. This is known as the multiple comparisons problem. Methods like Bonferroni correction exist to adjust for this increased risk.
Factors Influencing Type I Error Rate
- Sample Size: Smaller sample sizes increase the likelihood of a Type I error.
- Data Variability: High variability in the data can make it harder to distinguish real effects from random noise, increasing the chance of a Type I error.
- Test Selection: Choosing an inappropriate statistical test can increase the risk of Type I errors.
- Researcher Bias: Unconscious biases can influence data interpretation and increase the likelihood of making a Type I error.
FAQ: Decoding Type I Error
What does a Type I error mean in plain English? It means you've found something significant when nothing is really there. You've falsely concluded there's an effect or difference.
How does it influence the reliability of research findings? Type I errors lead to unreliable and potentially misleading conclusions. They can waste resources and lead to incorrect decisions.
Is it always easy to detect a Type I error? No. Detecting a Type I error is often difficult because it requires knowing the true state of the null hypothesis.
What are the consequences of ignoring Type I errors? Ignoring Type I errors can lead to incorrect conclusions, wasted resources, and potentially harmful decisions.
How can we minimize the risk of Type I errors? We can minimize the risk by using appropriate statistical methods, choosing adequate sample sizes, and being aware of potential biases.
Practical Tips to Master Understanding and Minimizing Type I Error
Start with the Basics: Understand the definition of the null hypothesis and the concept of a p-value.
Step-by-Step Application: Learn how to conduct hypothesis tests and interpret p-values correctly.
Learn Through Real-World Scenarios: Practice identifying potential Type I errors in different contexts.
Avoid Pitfalls: Be aware of the multiple comparisons problem and use appropriate corrections.
Think Creatively: Consider alternative explanations for significant results before drawing conclusions.
Go Beyond: Explore advanced statistical methods for controlling Type I error rates and understand the role of power analysis in study design.
Conclusion: Type I error is more than just a statistical concept; it's a crucial element in understanding the reliability of our research and the validity of our conclusions. By mastering its nuances, you unlock the ability to make more informed decisions based on sound statistical analysis, enhancing the integrity of research across diverse fields.
Closing Message: Embrace a cautious yet critical approach to statistical interpretation. By understanding the pitfalls of Type I error and employing the appropriate safeguards, you empower yourself to navigate the complexities of data analysis and draw robust, reliable conclusions that are grounded in evidence and free from the deception of false positives. The pursuit of accurate knowledge requires a thorough understanding and respect for the potential pitfalls inherent in statistical inference.
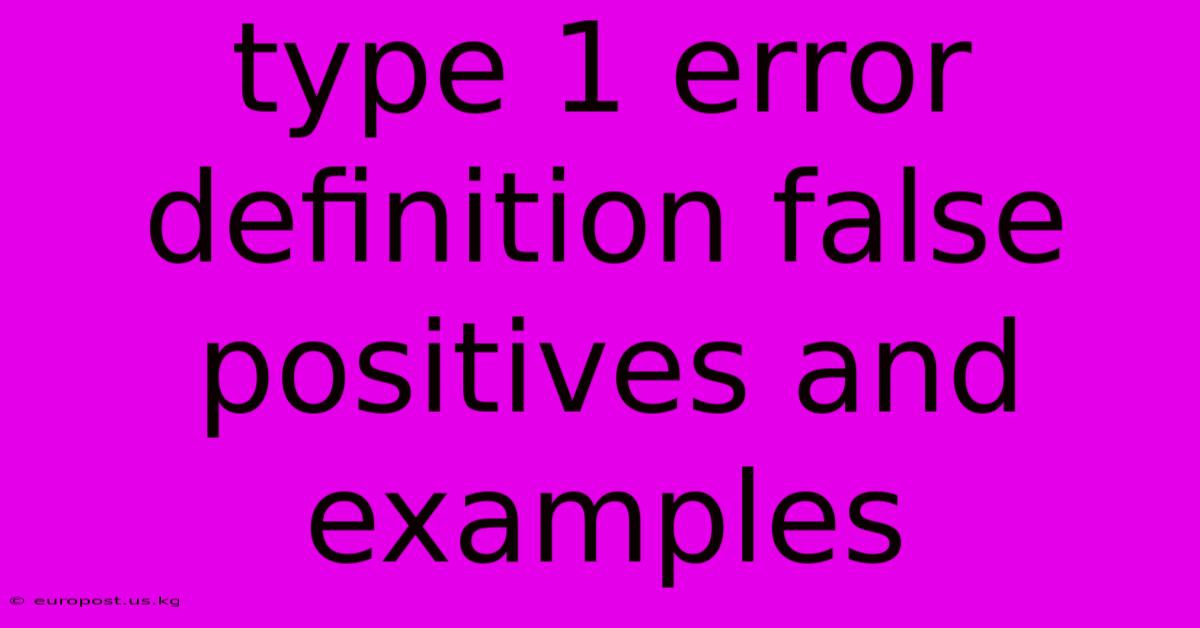
Thank you for taking the time to explore our website Type 1 Error Definition False Positives And Examples. We hope you find the information useful. Feel free to contact us for any questions, and don’t forget to bookmark us for future visits!
We truly appreciate your visit to explore more about Type 1 Error Definition False Positives And Examples. Let us know if you need further assistance. Be sure to bookmark this site and visit us again soon!
Featured Posts
-
Tax Deductible Interest Definition And Types That Qualify
Jan 20, 2025
-
What Is Umbrella Insurance Policy Definition And If You Need It
Jan 20, 2025
-
What Are Tranches Definition Meaning And Examples
Jan 20, 2025
-
Hammering Definition
Jan 20, 2025
-
Trade Resumption Definition
Jan 20, 2025