What Is A Two Tailed Test Definition And Example
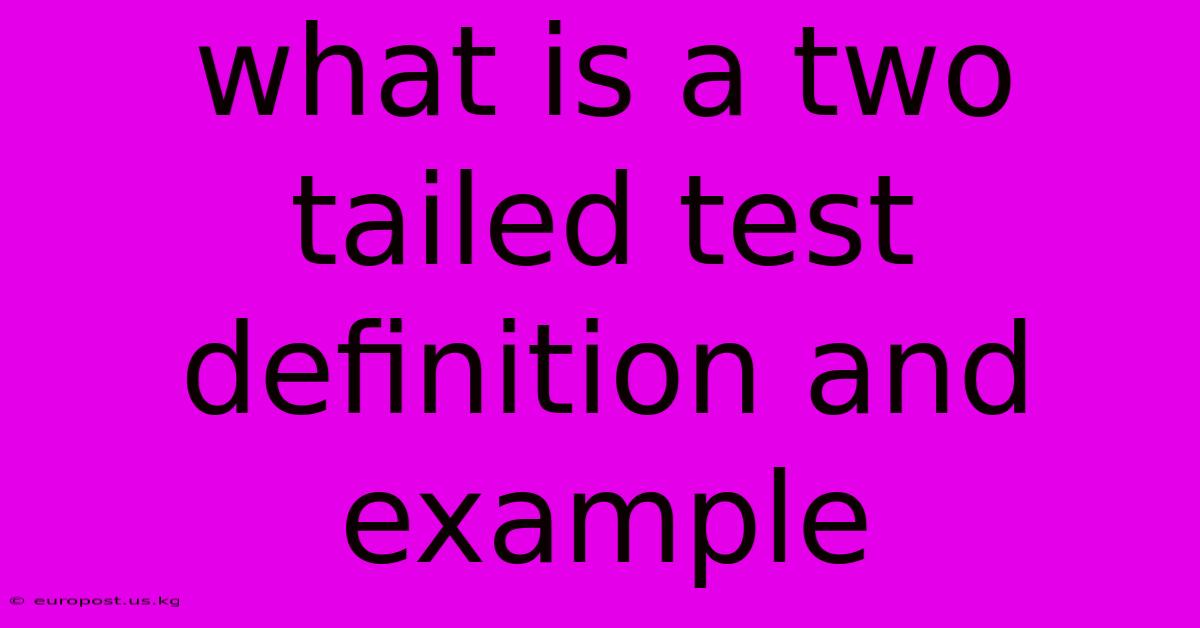
Discover more in-depth information on our site. Click the link below to dive deeper: Visit the Best Website meltwatermedia.ca. Make sure you don’t miss it!
Table of Contents
Unveiling the Secrets of the Two-Tailed Test: Exploring Its Pivotal Role in Hypothesis Testing
Introduction: Dive into the transformative power of the two-tailed test and its profound influence on statistical analysis and decision-making. This detailed exploration offers expert insights and a fresh perspective that captivates statisticians, researchers, and data analysts alike.
Hook: Imagine needing to determine if a new drug significantly affects blood pressure. A one-tailed test might only check if the drug lowers blood pressure, ignoring the possibility it raises it. A two-tailed test, however, provides a more comprehensive assessment, investigating whether the drug causes a significant change in either direction. This seemingly small difference has huge implications for the accuracy and reliability of your conclusions.
Editor’s Note: A groundbreaking new article on two-tailed tests has just been released, uncovering its essential role in shaping accurate and robust statistical inferences.
Why It Matters: The two-tailed test is a cornerstone of statistical hypothesis testing, allowing researchers to investigate whether a sample mean differs significantly from a population mean without pre-conceived notions about the direction of that difference. This impartiality is crucial for maintaining objectivity and avoiding biased interpretations of data. Understanding its nuances is vital for drawing valid conclusions from experimental data across diverse fields, from medicine and engineering to economics and social sciences.
Inside the Article
Breaking Down the Two-Tailed Test
Purpose and Core Functionality: The primary purpose of a two-tailed test is to determine if there's a statistically significant difference between a sample statistic (like the mean) and a hypothesized population parameter. Unlike a one-tailed test, which focuses on a specific direction (e.g., greater than or less than), the two-tailed test examines deviations in both directions. This means it tests for the possibility that the sample mean is either significantly greater than or significantly less than the hypothesized population mean.
Role in Hypothesis Testing: The two-tailed test fits neatly into the framework of null hypothesis significance testing (NHST). The null hypothesis (H₀) typically states there is no significant difference between the sample and population means. The alternative hypothesis (H₁) is that there is a significant difference, without specifying the direction. The test statistic calculated from the sample data is compared to a critical value determined by the significance level (alpha, often 0.05) and the degrees of freedom. If the test statistic falls within the critical regions (in both tails of the distribution), the null hypothesis is rejected.
Impact on Significance Levels and p-values: The significance level (alpha) is divided equally between the two tails of the distribution in a two-tailed test. For example, with alpha = 0.05, each tail will have an area of 0.025. The p-value, representing the probability of observing the obtained results (or more extreme) if the null hypothesis were true, is doubled in a two-tailed test. This is because the p-value reflects the probability in both tails.
Exploring the Depth of the Two-Tailed Test
Opening Statement: What if you needed an unbiased way to assess the impact of a new teaching method on student scores? A two-tailed test provides precisely that. It allows you to evaluate whether the new method leads to a significant improvement or a significant decline in scores, ensuring a fair and comprehensive analysis.
Core Components: The core components of a two-tailed test include:
- Sample Data: The collected data from your experiment or study.
- Hypotheses: The null (H₀) and alternative (H₁) hypotheses, clearly stating the claims being tested.
- Test Statistic: A calculated value (e.g., t-statistic, z-statistic) based on the sample data.
- Significance Level (alpha): The probability of rejecting the null hypothesis when it is actually true (Type I error).
- Critical Values: The values that define the boundaries of the rejection regions in the distribution.
- Degrees of Freedom: A value that depends on the sample size and the type of test being used.
- P-value: The probability of observing the obtained results (or more extreme) if the null hypothesis were true.
In-Depth Analysis: Consider a study investigating the effect of a new fertilizer on crop yield. The null hypothesis is that there's no difference in yield between the control group (using the old fertilizer) and the experimental group (using the new fertilizer). A two-tailed test would assess whether the new fertilizer significantly increases or decreases yield. A significant p-value (less than alpha) would lead to rejecting the null hypothesis, indicating a statistically significant difference in either direction.
Interconnections: The two-tailed test's power is significantly influenced by the sample size. Larger samples provide more precise estimations and increase the test's ability to detect a true difference. The choice between a one-tailed and a two-tailed test is crucial and depends on the research question and prior knowledge. If there's a strong theoretical reason to expect a difference in a specific direction, a one-tailed test might be appropriate. However, two-tailed tests are generally preferred due to their greater objectivity and robustness.
FAQ: Decoding the Two-Tailed Test
What does a two-tailed test do? It assesses whether a sample mean differs significantly from a hypothesized population mean in either direction (greater than or less than).
How does it influence conclusions? It provides a more comprehensive and unbiased evaluation, preventing potentially misleading conclusions drawn from focusing on a single direction.
Is it always relevant? While one-tailed tests have their place, the two-tailed test is often the more appropriate choice due to its broader applicability and protection against potential biases.
What happens when a significant difference is found? The null hypothesis is rejected, indicating that there's statistically significant evidence to support the alternative hypothesis, suggesting a difference exists.
What are the limitations? Statistical significance doesn't always imply practical significance. A small but statistically significant difference may not be meaningful in a real-world context.
Practical Tips to Master the Two-Tailed Test
Start with the Basics: Understand the core concepts of hypothesis testing, including null and alternative hypotheses, significance levels, and p-values.
Step-by-Step Application: Familiarize yourself with the steps involved in conducting a two-tailed test, from data collection and hypothesis formulation to test statistic calculation and p-value interpretation. Use statistical software packages (like R, SPSS, or Python's SciPy) to perform the calculations.
Learn Through Real-World Scenarios: Work through diverse examples across various fields to solidify your understanding.
Avoid Pitfalls: Be cautious of interpreting p-values in isolation. Consider effect size, sample size, and the context of the study when drawing conclusions.
Think Creatively: Apply your knowledge to real-world problems, designing appropriate tests for specific research questions.
Go Beyond: Explore advanced topics, such as power analysis, to better design studies and maximize the chances of detecting true effects.
Conclusion: The two-tailed test is more than a statistical tool—it’s a cornerstone of rigorous and unbiased scientific inquiry. By mastering its nuances, you unlock the ability to draw valid and reliable conclusions from data, improving the quality of research and decision-making across numerous disciplines.
Closing Message: Embrace the power of the two-tailed test to unlock new possibilities in data analysis and interpretation. Its inherent objectivity and comprehensive approach ensure more robust and reliable conclusions, leading to more informed decisions in your field of study. Remember to consider both potential directions of difference, thereby enhancing the accuracy and credibility of your findings.
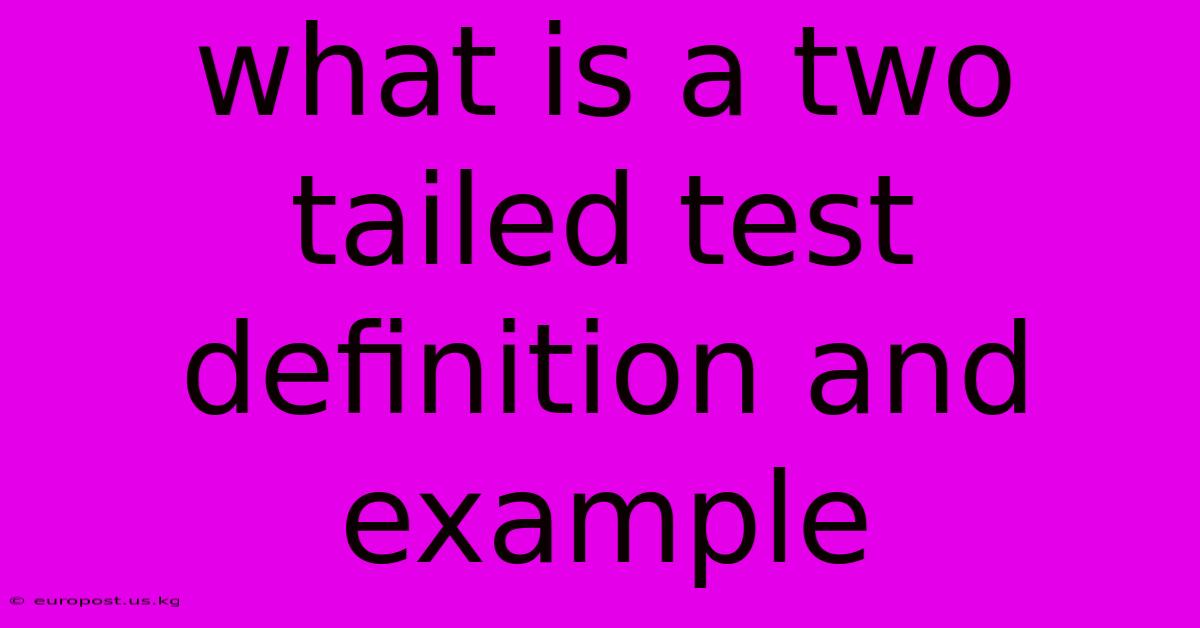
Thank you for taking the time to explore our website What Is A Two Tailed Test Definition And Example. We hope you find the information useful. Feel free to contact us for any questions, and don’t forget to bookmark us for future visits!
We truly appreciate your visit to explore more about What Is A Two Tailed Test Definition And Example. Let us know if you need further assistance. Be sure to bookmark this site and visit us again soon!
Featured Posts
-
Treasury Secretary Definition
Jan 20, 2025
-
What Is A Trustee Definition Role And Duties
Jan 20, 2025
-
Treasury Stock Treasury Shares Definition Use On Balance Sheets And Example
Jan 20, 2025
-
Growth Rates Formula How To Calculate And Definition
Jan 20, 2025
-
Initial Interest Rate Definition
Jan 20, 2025